April 02, 2025
AI has been in existence from long, but GenAI made it a coffee table conversation. Today it is impacting, even disrupting multiple sectors including BFSI. Traditional AI applications have already made significant inroads in areas like fraud detection and customer service chatbots. However, what is at the doorstep is fundamentally different – Agentic AI.
Agentic AI introduces the ability for AI systems to act autonomously, beyond task execution. These systems possess goal-directed behavior — meaning they can set objectives, plan multi-step tasks, adapt to changing environments, and execute workflows without explicit step-by-step instructions with minimal or no human intervention.
Agentic AI agents can combine planning, reasoning, and memory to pursue outcomes. In BFSI, Agentic AI offers the potential to rethink traditional workflows entirely.
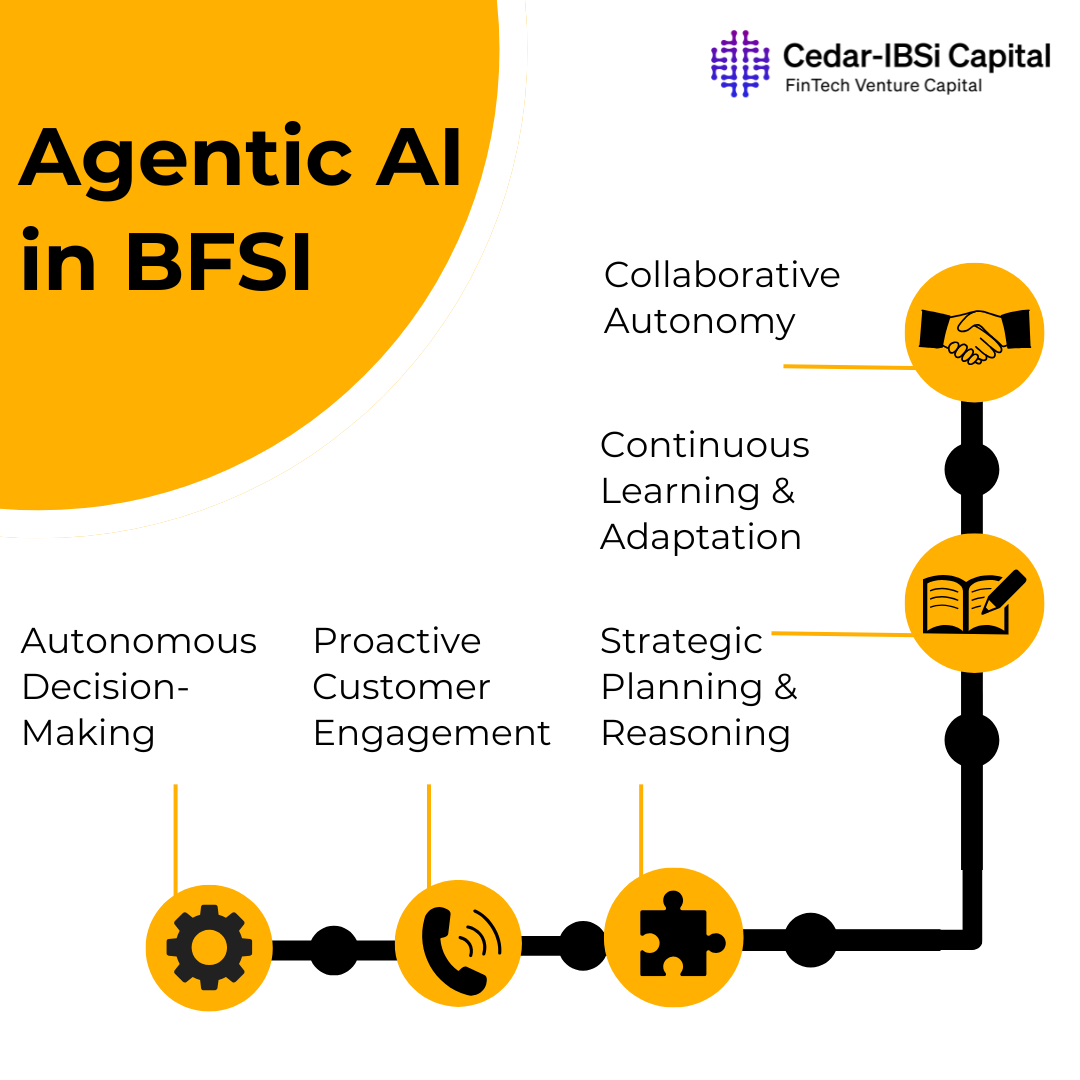
- Autonomy: AI agents can operate independently without constant human intervention. They can initiate tasks, make decisions, and take actions based on their understanding of the environment and their defined objectives. For example, an agent monitoring market trends could autonomously execute trades based on predefined risk parameters.
- Proactivity: Unlike passive AI systems, agents can anticipate future needs and proactively take steps to address them. A customer service agent could proactively reach out to a customer experiencing difficulties with a transaction before the customer even raises a complaint.
- Reasoning and Planning: Agentic AI possesses the ability to reason through complex problems, develop multi-step plans, and adapt those plans in response to changing circumstances. This allows them to tackle intricate tasks that require strategic thinking, such as designing personalized financial plans.
- Learning and Adaptation: AI agents can learn from their experiences, refine their strategies, and improve their performance over time. Through continuous learning, they can adapt to evolving market conditions, customer preferences, and regulatory landscapes.
- Interaction and Collaboration: Agentic AI can interact with other agents, systems, and even humans to achieve their goals. This collaborative capability enables the creation of sophisticated workflows and the automation of complex, multi-stakeholder processes.
Agentic AI Use Cases Across BFSI Sub-Sectors
- Banking & Retail Finance
- Use Case: Autonomous Loan Origination
Today, AI-powered credit scoring is widely used. Agentic AI can extend this to autonomously handle loan application intake, documentation checks, compliance verification, and dynamic pricing — all in real-time.
For instance, an Agentic AI agent could adjust lending terms automatically based on updated borrower profiles or market conditions, without waiting for human intervention. - Example:
In the US, Wells Fargo and JPMorgan Chase are experimenting with agent-based AI systems to automate loan servicing workflows beyond just credit decisions, focusing on reducing turnaround times and improving personalization.
- Use Case: Autonomous Loan Origination
- Capital Market
- Use Case: Autonomous Trading Agents
Agentic AI can optimize portfolio rebalancing, liquidity management, and real-time trading decisions. Traditional AI might execute a trade based on a signal; Agentic AI can manage an entire portfolio objective (e.g., maximize yield while minimizing risk) by coordinating multiple agents analyzing market data, news, and macroeconomic trends. - Example:
BlackRock is piloting AI agents in its Aladdin platform to manage portfolio risk autonomously under pre-defined risk thresholds, allowing for dynamic adjustments in response to market movements (Bloomberg, 2023).
- Use Case: Autonomous Trading Agents
- Insurance
- Use Case: End-to-End Claims Processing
Beyond simple claims triage, Agentic AI agents could autonomously assess claims, request missing information, negotiate settlements, and flag suspected fraud.
Agentic AI promises to bring further reductions by acting without needing step-by-step human guidance. - Example:
Leading insurers in Europe are testing agent-based systems for low-value auto and property claims, achieving faster settlements and improved customer satisfaction.
- Use Case: End-to-End Claims Processing
- Compliance & Risk Management
- Use Case: Autonomous Regulatory Monitoring
Global BFSI players face thousands of regulatory updates annually. Agentic AI agents can autonomously track regulatory changes, analyze their implications on internal policies, and propose (or even implement) policy adjustments. - Example:
European banks piloting autonomous agents for MiFID II compliance saw up to 25% faster implementation of new regulatory requirements compared to teams relying solely on human compliance analysts.
- Use Case: Autonomous Regulatory Monitoring
Challenges Ahead
Despite the potential, it’s a long road ahead leading to mature Agentic AI in BFSI.
- Explainability
Agentic AI agents operate autonomously and may not follow linear, rule-based logic. This creates challenges for auditors, regulators, and internal governance teams seeking to understand how decisions were made. Transparent models and AI governance frameworks will become increasingly critical. - Regulatory Uncertainty
Most global regulatory frameworks, including the EU AI Act and guidelines from US and Asian regulators, currently address traditional AI. The introduction of autonomous agents will prompt regulators to revisit themes like accountability, auditability, and operational resilience. - Operational Risk
Autonomous agents acting independently could, if not properly governed, make sub-optimal or even harmful decisions. Banks and insurers will need to carefully manage this risk through human-in-the-loop systems, where agents operate autonomously but within defined guardrails and with oversight. - Talent & Readiness
Developing, deploying, and governing Agentic AI requires expertise in AI engineering, risk management, and BFSI domain knowledge — a combination that remains scarce and will take time to develop.
The Road Forward
The adoption of Agentic AI in BFSI is inevitable but will likely be progressive rather than immediate. Leading institutions are starting with:
- Narrowly scoped pilots: Focused on specific processes such as regulatory reporting, simple lending products, or claims servicing.
- Hybrid AI systems: Combining autonomous agents with human oversight, particularly for high-risk decisions.
- Enhanced AI governance: Strengthening model risk management frameworks to include explainability, auditability, and accountability for autonomous agents.
In the next 3–5 years, we are likely to see Agentic AI used most extensively in:
- Retail banking: End-to-end digital lending and customer service.
- Capital markets: Dynamic portfolio and liquidity management.
- Insurance: Autonomous low-value claims processing and fraud detection.
- Compliance: Real-time regulatory monitoring and automated policy adjustments.
The BFSI sector has always been cautious when adopting new technologies — and for good reason. However, given the potential of Agentic AI to transform decision-making and operational agility, financial institutions that move early and carefully will be well-positioned for the next phase of AI-driven transformation.